
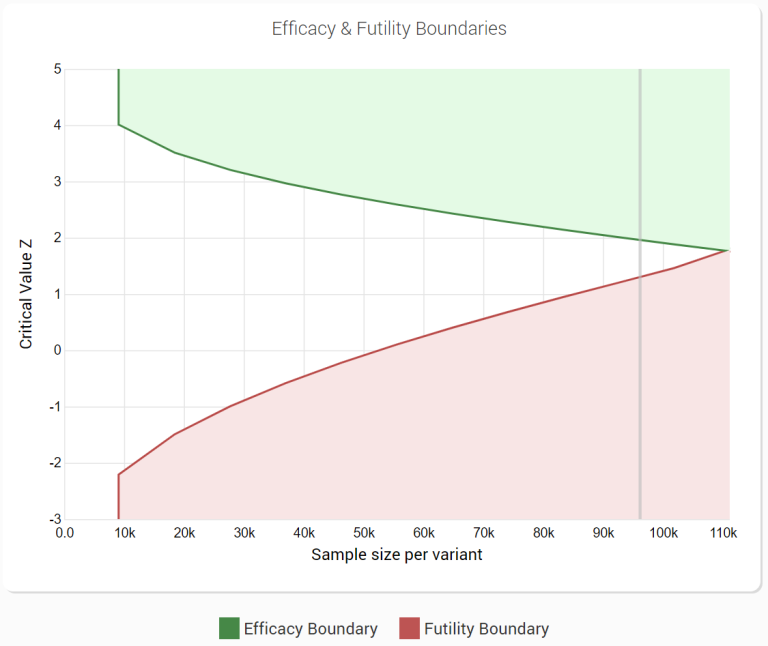
We cannot stop a test and refute the null hypothesis as soon as the p-Value is below the Significance Level. Thus we’ll be more likely to refute an actually true hypothesis.
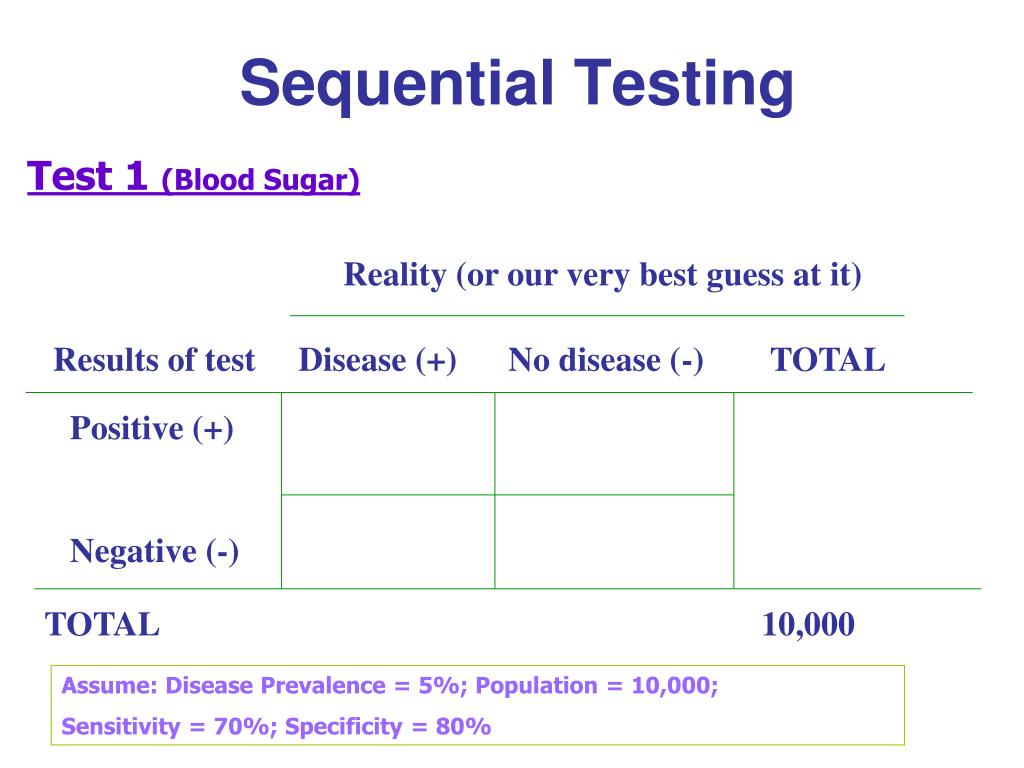
With peeking, we will end up with nominal p-Values much smaller than the actual ones. Hence the Type-I-Error rate of our test increases every time we have a look at our results! If instead, we check the results k times while conducting the test, we have k opportunities to make a wrong decision based on the results. The minimum required sample size is calculated so that when we have enough samples, the probability to falsely refute the null hypothesis when looking at the results is the selected level of significance. We would spend a lot of money for nothing! Peeking and the False-Positive Rate (or Type-I-Error)Ī test’s false positive rate is based on the assumption that we check the results only once, after obtaining enough samples. Imagine we wrongly conclude that a costly campaign had a positive effect on our test users and now we roll it out to everyone else. It is crucial in AB-tests to control the probability that we make a wrong decision and reject the null hypothesis although it is true. So if we set our significance level on say 5%, the likelihood that we falsely infer that our new design, campaign or feature has a real effect is not bigger than 5%.Ĭontrolling the risk of wrongly rejecting the null hypothesis is crucial in AB-testing. The significance level determines the probability that we wrongly conclude that there is a real effect in our experiment. When setting up an experiment, we set our desired false-positive rate (or Type-I-Error rate) using the significance level. Unfortunately, continuously monitoring our test statistics (or peeking) increases the probability to refute the null hypothesis although there’s no real effect. Monitoring the p-Value over time (Image by Author) We would reject the null hypothesis every time we peek and the p-Value hits the significance threshold (marked by the red dots): Let’s assume we peek 8 times before we obtain the required sample size n, depicted by the black and red dots.
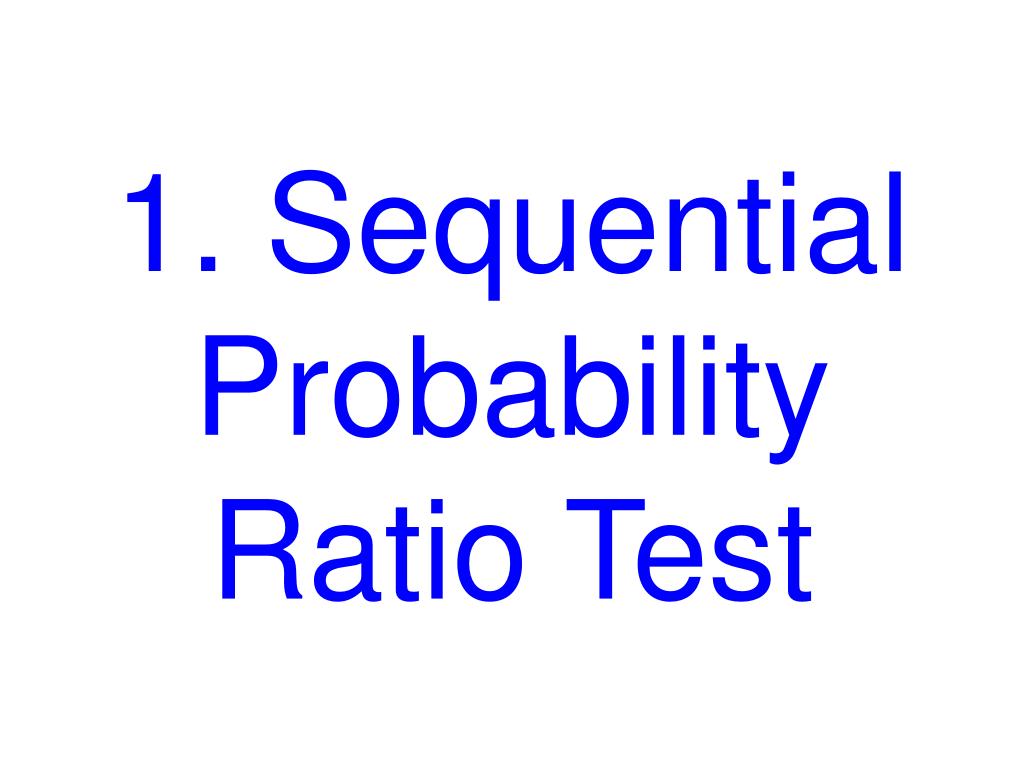
The p-Value fluctuates over time as we get more samples. One might be tempted to monitor the p-Value continuously and refute the null hypothesis as soon as it is below our significance threshold. Calculate the p-value and reject the null hypothesis if the p-value is below the chosen significance threshold.Run the test and collect n samples per test cell.Calculate the Minimum Required Sample Size n.Set parameters such as Significance Level, Power Level and Minimum Detectable Effect.This is due to the nature of fixed sample size tests and how they are conducted: Not checking the results before the minimum required sample size is obtained is one of the fundamental principles of AB-testing. In both cases ending the experiment early would have a positive effect on our revenue. If on the other hand, the design compels more users to finish their purchase, we would also like to stop the test as early as possible so that all users can be exposed to the new design. In case the design harms the Conversion Rate, we would like to stop the experiment as early as possible to prevent any further losses in revenue. Let’s assume we are testing a new design on our website’s checkout page. We want to peek to minimize the harm of bad tests and maximize the benefits from good test cells. Monitoring an AB-Test in Optimizely (Image by Author) Why we want to peekĬollecting enough samples can take weeks or even months, and there are good reasons why we want to check our results earlier (also known as peeking).
